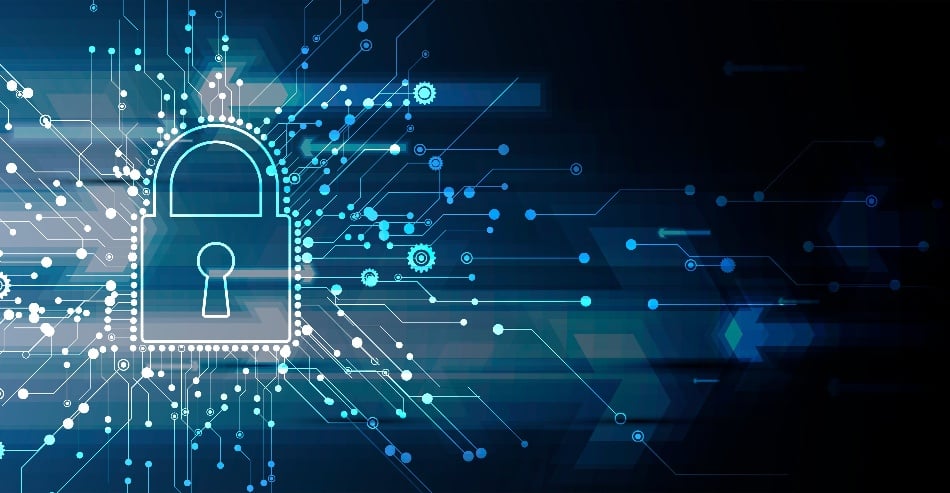
Our world is awash in data. The ubiquity of digital technology from smartphones to smart sensors means a constant and growing stream of data that has expanded beyond the capacity of humans to process using traditional methods.
AI allows us to derive actionable insights from massive data sets at an unprecedented speed and scale.
One area where these abilities are proving transformative is risk management. In this blog, we’ll explore the benefits of AI in risk management and how its sophisticated methodologies are shaping a safer tomorrow.
Learn more about the impact risk management has on our world in our interactive resource: The Future of Risk Analysis, Risk Engineering, and Risk Management: Machine Learning, Uncertainty Analysis, and Digital Twins.
Defining AI and Machine Learning for Risk Management
Let’s begin with an overview of AI and machine learning and why they are useful in the field of risk management.
Artificial Intelligence (AI) represents a field of computing that creates systems designed to mimic human intelligence. Machine learning is a branch of this field that uses algorithms to process data in a way that is capable of gradual improvement as the system “learns” through feedback. Machine learning is primarily used to detect patterns and make predictions.
These technologies are particularly useful when it comes to unstructured data. This is data in its raw form before it has been organized in some way by a data analyst. In the past, this kind of data had to first be categorized before being analyzed. Machine learning, however, can uncover useful patterns in unstructured collections without human intervention.
Approximately 80-90% of all data generated is unstructured. We simply don’t have the capacity to manually organize all the digital data we are creating. Yet it is often in that unstructured data that the most important information is hiding.
This is certainly true in the realm of risk management. The world is incredibly complex and we need to be able to deal with both known risks as well as emerging risks that are not yet easily visible. By being able to analyze unstructured data, AI and machine learning for risk management provides an unparalleled advantage in terms of rapidly analyzing data and creating actionable insights from it.
It helps those working in risk management analyze massive amounts of unstructured data at scale to identify patterns and make predictions about known and unknown risks.
Concrete Uses of AI in Risk Management
AI is a useful tool that strengthens our ability to identify and mitigate risks, allowing those working in this field to make better and more timely decisions.
Here are three ways AI and machine learning is being used for risk management today:
Threat analysis and risk assessment
Machine learning can extract important insights from data about potential risks and their probability.
For example, machinery in areas like manufacturing and mining is often monitored by an array of sensors. AI can identify patterns associated with malfunction to allow for an accurate assessment of equipment health in real-time and proactive maintenance to prevent breakdown.
Risk reduction
Using AI and machine learning, engineers can discover patterns associated with past problems to predict future risks and design systems and procedures to avoid them.
This is an important strategy in cybersecurity. Insights from past user behavior associated with security breaches allow companies to put in place policies and safeguards to reduce the risk of the same kind of breach happening again in the future.
Data classification
Machine learning excels at grouping unstructured data into meaningful sets based on similarities that can be complex and difficult for humans to spot. It can also easily group data based on predefined categories.
In either case, the end result is data that is more organized, more accessible and more useful in the effort to identify and control risks.
Three Benefits of AI and Machine Learning for Risk Management
The use of AI and machine learning for risk management enables improvements over traditional methods in three key areas:
- Greater efficiency: Gathering, categorizing and processing data used to be a labor- and time-intensive process. Not only can machine learning handle much more data, but it can do so using fewer resources.
- Improved timeliness: The best time to know about the presence of risk is now. AI enables real-time monitoring and analysis so we can identify and respond to risks much more quickly than was possible before.
- Improved data processing: The ability to analyze unstructured data on a large scale is a significant advance. We can now uncover significant patterns and make predictions that would have been impossible a few years ago.
The Future of Risk Management is Being Shaped by AI
Just as in many other industries, the adoption of AI and machine learning is profoundly changing the practice of risk management.
We can expect this transformation to continue into the future as the growth of the Internet of Things (IoT) and our reliance on digital technology will produce more data about more areas of human activity.
The greater the complexity of a system, the more can go wrong and the harder it is to adequately monitor it. In an increasingly interconnected world, we will rely more than ever on the ability of computers to help us quickly identify and predict risks to prevent critical system failures.
Vanderbilt Alumni Are Leading the Charge in Developing New Risk Management Methodologies with AI
Students in Vanderbilt School of Engineering’s Risk, Reliability, and Resilience program learn about the latest techniques in machine learning and how to leverage their abilities in the area of risk management.
Recent Civil Engineering PhD program alumnus, Xiaoge Zhang, developed an algorithm to increase safety on the ground at airports. Surface operations have a lot of moving parts, from aircraft to fuel trucks to baggage trains. Collisions can lead to injury to passengers or airport personnel and damage to equipment.
To help reduce the risks associated with all this activity, Zhang and fellow researchers used machine learning to predict the movements of surface vehicles based on past patterns and provide an assessment of collision risks.
Help Shape a Safer Future with AI by Earning Your Master of Engineering in Risk, Reliability and Resilience
Using the power of AI as an engineer to help create systems and structures that are safer and less prone to failure is an exciting opportunity. In the years ahead, this field will make a decisive impact in areas including healthcare, infrastructure, defense, manufacturing, cybersecurity, energy/environment and transportation.
At Vanderbilt School of Engineering, the RRR program is uniquely designed to equip students for leadership in helping organizations in any of these areas properly account for uncertainty and risk and develop ways to improve quality, efficiency, safety and protection of the environment.
If you’d like to find out more about how Vanderbilt’s M.Eng. degree in Risk, Reliability and Resilience can open doors for you in the field of risk management, you can view our program requirements and request more information.
For more insights like this one about the latest trends in engineering, subscribe to our blog, the EngineeringVU
Ready to make your mark on risk management at Vanderbilt School of Engineering? Apply today!